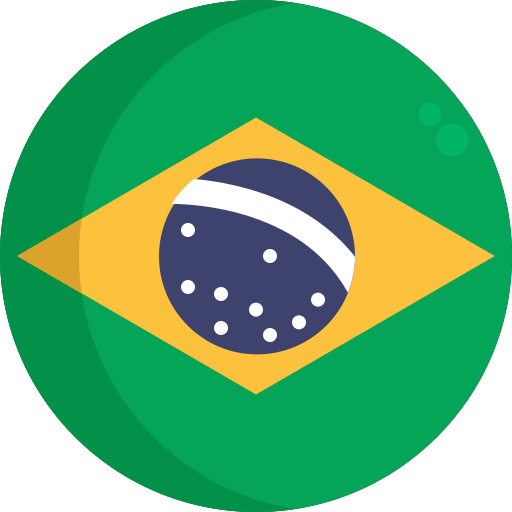
Speeding Up Recovery from Concept Drifts
2014; Springer Science+Business Media; Linguagem: Inglês
10.1007/978-3-662-44845-8_12
ISSN1611-3349
AutoresSilas Garrido Teixeira de Carvalho Santos, Paulo Mauricio Gonçalves Júnior, Geyson Daniel dos Santos Silva, Roberto Souto Maior de Barros,
Tópico(s)Advanced Bandit Algorithms Research
ResumoThe extraction of knowledge from data streams is an activity that has progressively been receiving an increased demand. However, in this type of environment, changes in data distribution, or concept drift, can occur constantly and is a challenge. This paper proposes the Adaptable Diversity-based Online Boosting (ADOB), a modified version of the online boosting, as proposed by Oza and Russell, which is aimed at speeding up the experts recovery after concept drifts. We performed experiments to compare the accuracy as well as the execution time and memory use of ADOB against a number of other methods using several artificial and real-world datasets, chosen from the most used ones in the area. Results suggest that, in many different situations, the proposed approach maintains a high accuracy, outperforming the other tested methods in regularity, with no significant change in the execution time and memory use. In particular, ADOB was specially efficient in situations where frequent and abrupt concept drifts occur.
Referência(s)