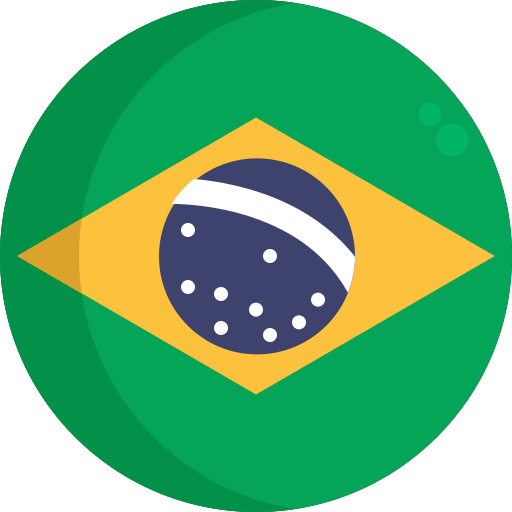
Forecasting Daily Emergency Department Visits Using Calendar Variables and Ambient Temperature Readings
2013; Wiley; Volume: 20; Issue: 8 Linguagem: Inglês
10.1111/acem.12182
ISSN1553-2712
AutoresIzabel Marcílio, Shakoor Hajat, Nélson Gouveia,
Tópico(s)Healthcare Policy and Management
ResumoThis study aimed to develop different models to forecast the daily number of patients seeking emergency department (ED) care in a general hospital according to calendar variables and ambient temperature readings and to compare the models in terms of forecasting accuracy. The authors developed and tested six different models of ED patient visits using total daily counts of patient visits to an ED in Sao Paulo, Brazil, from January 1, 2008, to December 31, 2010. The first 33 months of the data set were used to develop the ED patient visits forecasting models (the training set), leaving the last 3 months to measure each model's forecasting accuracy by the mean absolute percentage error (MAPE). Forecasting models were developed using three different time-series analysis methods: generalized linear models (GLM), generalized estimating equations (GEE), and seasonal autoregressive integrated moving average (SARIMA). For each method, models were explored with and without the effect of mean daily temperature as a predictive variable. The daily mean number of ED visits was 389, ranging from 166 to 613. Data showed a weekly seasonal distribution, with highest patient volumes on Mondays and lowest patient volumes on weekends. There was little variation in daily visits by month. GLM and GEE models showed better forecasting accuracy than SARIMA models. For instance, the MAPEs from GLM models and GEE models at the first month of forecasting (October 2012) were 11.5 and 10.8% (models with and without control for the temperature effect, respectively), while the MAPEs from SARIMA models were 12.8 and 11.7%. For all models, controlling for the effect of temperature resulted in worse or similar forecasting ability than models with calendar variables alone, and forecasting accuracy was better for the short-term horizon (7 days in advance) than for the longer term (30 days in advance). This study indicates that time-series models can be developed to provide forecasts of daily ED patient visits, and forecasting ability was dependent on the type of model employed and the length of the time horizon being predicted. In this setting, GLM and GEE models showed better accuracy than SARIMA models. Including information about ambient temperature in the models did not improve forecasting accuracy. Forecasting models based on calendar variables alone did in general detect patterns of daily variability in ED volume and thus could be used for developing an automated system for better planning of personnel resources. Este estudio tiene el objetivo de desarrollar diferentes modelos para predecir el número diario de pacientes que buscan atención en el servicio de urgencias (SU) en un hospital general según las variables del calendario y las lecturas de la temperatura ambiente, así como comparar los modelos en términos de certeza predictiva. Se desarrollaron y comprobaron seis modelos diferentes de visitas de pacientes al SU mediante la suma diaria total de las visitas de los pacientes a un SU de Sao Paulo, Brasil, desde el 1 de enero de 2008 al 31 de diciembre de 2010. Los datos de los primeros 33 meses se usaron para desarrollar los modelos de predicción de visitas de pacientes al SU (el entrenamiento del modelo), y se dejaron los últimos tres meses para medir la certeza predictiva de cada modelo por el error porcentual absoluto medio. Los modelos pronósticos se desarrollaron mediante tres métodos de análisis de series temporales diferentes: modelos lineales generalizados, ecuaciones de estimación generalizadas, y modelos autorregresivos integrados de media móvil estacional (SARIMA). Para cada método, los modelos se exploraron con y sin el efecto de la media de temperatura diaria como una variable predictiva. La media del número de visitas diarias al SU fue de 389, con un rango de 166 a 613. Los datos mostraron una distribución estacional semanal, con los volúmenes de pacientes más altos los lunes y los más bajos en fines de semana. Hubo poca variación en las visitas diarias por mes. Los modelos lineales generalizados y los modelos de ecuación de estimación generalizada mostraron mejor certeza predictiva que los modelos SARIMA. Por ejemplo, el error porcentual absoluto medio de los modelos lineales generalizados y de los modelos de ecuaciones de estimación generalizadas en el primer mes de predicción (octubre, 2012), fue de 11,5% y 10,8% (modelos con y sin control para el efecto de la temperatura, respectivamente) mientas que los errores porcentuales absolutos medios de los modelos SARIMA fueron de 12,8% y 11,7% (respectivamente). Para todos los modelos, el control por el efecto de la temperatura resultó en una capacidad predictiva similar o peor que los modelos con sólo las variables del calendario, y la certeza predictiva fue mejor para el corto plazo (anterior a 7 días) que para el más largo plazo (anterior a 30 días). Este estudio indica que los modelos de series temporales pueden ser desarrollados para proporcionar predicciones de las visitas diarias de los pacientes al SU. La capacidad predictiva fue dependiente del tipo de modelo empleado y la duración del tiempo predicho. En este sentido, los modelos lineales generalizados y los modelos de ecuación estimativa generalizada mostraron mejor certeza. La inclusión de la información sobre la temperatura ambiente en los modelos no mejoró la certeza pronostica. Los modelos de predictivos basados únicamente en las variables del calendario en general detectaron patrones de variabilidad diaria en el volumen del SU, y por ello podrían ser usados para desarrollar un sistema automatizado para la mejor planificación de los recursos de personal. Reports from different countries, including the United States, the United Kingdom, and Brazil, have shown an increase in demand for emergency department (ED) care, resulting in frequently overcrowded EDs, lengthy waiting times for assistance, and an overall perception by patients of poor health care.1-4 Prolonged waiting times are described as a major factor for dissatisfaction with ED care,5, 6 and patients are more likely to leave without being seen as waiting time increases.5 While common practice is to divert patients from EDs in times of overcrowding,2 using data on daily patient volume for better planning of personnel resources might increase ED efficiency, as well as improve ED patient care quality.5, 7-9 A report from the National Audit Office on inpatient admissions from acute hospitals in the United Kingdom has stated that the trusts could make more effective use of their knowledge of patterns of ED admissions to assess the likely demand for their resources.10 A time series is a set of chronologically ordered observations, and forecasting methods use past values of any given time series to predict its future behavior.11 Time-series models can be used to forecast future ED patient visits based on the estimated effect of predictor variables, and such forecasts can be used for proactive bed and staff management and for facilitating patient flow.12, 13 For example, the finding that Sundays had a much higher volume of patients in the ED at a hospital in Israel led to the decision of allocating an additional physician to staff every Sunday, thus alleviating ED congestion.9 Batal et al.5 have reported an 18.5% decrease in patients leaving without being seen in an ED and a 30% decrease in complaints after adjusting staff in accordance to the results of an applied ED patient visits forecasting model. Although decisions on staffing are commonly based on personal experience,14-16 a rational approach to allocation of resources would be of great importance for improving the quality of care delivered in EDs.1-3, 5, 17 A number of factors can influence daily ED visits, and a patient's visits forecasting model should include those factors. Previous studies have shown that ED visits present cyclical variations according to calendar variables, such as day of the week, time of the year, and the occurrence of public holidays.1, 5, 9, 12, 13, 17 Temperature variables have also been included in some patient visits forecasting models,14, 17-20 because many studies have demonstrated the association of climate factors, temperature in particular, with the occurrence of mortality and morbidity outcomes.21-24 For instance, weather forecasts in the United Kingdom have been used for warning chronic obstructive pulmonary disease patients when their health is likely to be affected.25 The predictive effect of temperature on daily ED visits, however, is still uncertain. While some studies have shown there is an association between these variables,12, 17-20, 26 other authors advocate that including temperature adds uncertainty to the model in exchange for little improvement on forecasting accuracy.5, 12, 27 Because the temperature effect depends on the geographical location and on characteristics of the ED,26 the relevance of including weather variables for improving the overall prediction accuracy should be tested when developing a particular forecasting model for daily ED visits. Our study aims to develop models to forecast the daily number of patients seeking ED care in a busy general hospital in a major world city (Sao Paulo, Brazil) according to calendar variables and ambient temperature. Different time-series approaches can be employed to develop forecast models, and the relevant literature indicates that there is no obvious supremacy of one method over others.11 We thus explored three different analytic approaches to develop daily ED patient visits forecasting models as well as the contribution of ambient temperature and compared the models in terms of forecasting accuracy. To develop and compare accuracy of forecast models of ED patient visits using different time-series analysis methods, we evaluated records of daily ED visits to a tertiary hospital. The study was approved by the Ethical Committee Review Board of the University of Sao Paulo Clinics Hospital. The study was conducted in Sao Paulo, Brazil, a city of approximately 11 million people. The ED is the main referral hospital for high-complexity emergency clinical, surgery, and trauma cases occurring in the south and west region of the city. Operating 7 days per week, 24 hours a day, the ED treats approximately 180,000 patients per year. Data on daily ED patient visits, including date and time of arrival and main diagnosis, were extracted from a computerized tracking system at the hospital's information and health department. We extracted total daily counts of all patients who presented to the ED from January 1, 2008, to December 31, 2010. Daily mean temperature data for the study period were obtained from the Sao Paulo Environmental Agency. These environmental data are collected hourly at 12 fully automated monitoring stations throughout Sao Paulo. Mean temperature was calculated as an average of all 24-hour measurements at the 12 stations. The database of daily ED visits and ambient temperature was divided into two periods. The first period, from January 1, 2008, to September 30, 2010, was used for initial data analysis and model development (the "training set"). The second period, from October 1, 2010, to December 31, 2010, was used to apply the ED patient visits forecasting models and test their accuracy (the "postsample forecasting set"). The postsample forecasting set was further divided into three forecasting horizons of 1 month each (October, November, and December 2010), and forecast accuracy was assessed at horizons of 7 and 30 days in advance. After forecasting daily ED visits for the first horizon (October) and measuring the model's accuracy, the observed values of ED visits were incorporated into the training set and the model reestimated, with the resulting outputs being used to forecast ED visits for the second horizon (November). The process was then repeated for the third horizon (December). This 30-day horizon approach was chosen as we wanted to simulate a real case scenario in which the forecasting model could be updated with the observed values as time went by, and new forecasting values would be generated for future dates. We explored models of ED patient visits using three forecasting methods: generalized linear models (GLM), generalized estimating equations (GEE), and seasonal autoregressive integrated moving average (SARIMA) models. All analyses were conducted in Stata 12.0 (StataCorp, College Station, TX). Generalized linear models have been used widely in time-series regression studies of health outcomes in relation to environmental variables.21-24, 28 GEEs are an extension of GLMs that have been increasingly used for time-series analysis as well29-32 and provide the advantage of allowing for autocorrelation (nonindependence of ED patient visits on proximate days) to be taken into account in the postsample forecasting set.29, 30 We thus applied Poisson GLM and GEE models, allowing for overdispersion to quantify effects of the predictor variables on daily ED visits and to forecast the number of ED visits in the postsample forecasting set. For the GEE models, we considered autoregressive structures up to a 7-day lag, finally choosing an autoregressive structure of 1-day lag based on best model fit. An autoregressive time-series model is a multiple regression model in which the outcome variable is regressed on its past values,11 and the chosen 1-day lag means that the number of ED visits in one given day is mostly affected by the previous day's patient volume. Goodness of fit was assessed through comparison of quasi-likelihood under the independence model criterion (QIC).33 The third forecasting method we examined was a SARIMA model. Autoregressive integrated moving average (ARIMA) models describe current (and future) behavior of variables in terms of their past values and has been described as the most widely used in health events forecasting.3, 12, 17 SARIMA models extend basic ARIMA models and allow for the incorporation of seasonal patterns. In time-series analysis, seasonality refers to any repetitive pattern that occurs with a known periodicity, such as the weekly pattern observed in ED daily visits. A SARIMA model is typically represented by (p,d,q) (P,D,Q)s, where p represents the order of autoregression (AR), d is the order of differencing (i.e., data transformation through calculating differences among pairs of observations at a d lag to make a nonstationary series stationary), and q is the order of the moving average (MA). P, D, and Q are their seasonal counterparts, and s is the seasonal lag.11 Model identification was achieved by examining correlogram plots of the autocorrelation function (ACF) and partial autocorrelation function (PACF) of the data. These plots indicated that a SARIMA model of order (1,0,2) (1,1,2)7 had the best fit for our database, indicating an autoregressive structure of a 1-day lag, a moving average of a 2-day lag, and a weekly seasonality (a 7-day lag). Because there is some subjectivity in the interpretation of such plots,11 we compared alternative models based on Akaike information criterion (AIC), and the model described here was the model with the best fit. All ED patient visits forecasting models included calendar variables as predictors of ED visits. These calendar variables were day-of-week, public holidays (a total of 12 days per year), and the days before and after a holiday, because ED activity on such days can be affected by a rebound effect of the holiday. Because the H1N1 flu epidemic of 2009 produced an abnormal peak in ED visits in Sao Paulo due to respiratory causes,34 an indicator term for that period (July 13 to August 12, 2009) was included in the models. Moreover, because exceptionally low numbers of visits for the 31 of December and January first of each year were also identified, a term for these dates was also included in the models. Year-round seasonality was controlled for in all models by including Fourier terms, a data smoothing technique that uses sine–cosine functions for controlling of regular cyclical patterns in the data. Fourier terms were chosen because their specification does not depend on the actual data and thus can be used in the postsample forecasting equation. Long-term trend was accounted for by means of a linear term for date of admission. For each of the three forecasting methods, we tested one model with and one without temperature as a predictor of daily ED visits. Temperature has been shown to be the climate factor most consistently related to health outcomes.17, 22, 23, 26 Because health outcomes are associated with extremes of both heat and cold weather, temperature effect was modeled as a log linear increase below cold and above heat identified thresholds. Threshold values were determined by fitting models over all observed values in the temperature range and then selecting values with best model fit (lowest AIC). For ease of interpretation, the effect of each independent variable on the daily number of ED patient visits was expressed as the percentage change in daily patient volume. This measure of association is a conversion of the relative risk obtained from GLM and GEE models and represents the increase or decrease in the number of daily ED visits associated with each variable in relation to the reference category. Multivariate SARIMA models do not provide information on the overall effect of each parameter and thus was not used for effect estimation. Forecast accuracy was measured through comparison of plots showing the forecasted and observed values of daily ED visits and through calculation of the mean absolute percentage error (MAPE) in each horizon (7 and 30 days in advance) of the postsample forecasting set. MAPE is the mean of the absolute differences between forecasted and observed values expressed in terms of a percentage of the observed values; thus a lower MAPE reflects better forecasting accuracy. Being a scale-independent measure, MAPE can be used to compare forecasting results of different time-series models and other studies. We observed 390,192 ED patient visits during the training set period (1,004 days). The daily mean number of ED visits was 389, ranging from 166 visits to 613 visits. During the same period, daily mean ambient temperature was 19.5°C (fifth centile was 13.7°C, 95th centile was 24.5°C). The scatter plot of ED patient volume according to date of ED visit (Figure 1) across the study period shows an upward trend in visits over time, more evident from January 2009 onward. The plot also shows the different patterns of weekdays (higher patient volume) and weekend (lower patient volume) ED daily visits as evidenced by the two distinctive bands on the scatter plot throughout the study period. Box plots of the distribution of data by day-of-week and month (Figure 2) showed higher patient volumes on Mondays and lower volumes on weekends. However, there was little variation in daily visits by month. Table 1 shows the estimated effect of each predictor variable on daily ED visits obtained from the GLMs with and without terms for temperature and expressed in terms of percentage change in daily volume. Threshold values for cold and heat effects were both 16°C, and optimum lags were up to 20 and 5 days, respectively. Although temperature was associated with daily ED visits, with the cold effect being stronger than the heat effect, controlling for temperature did not change the estimated effect of the calendar variables. The effects obtained through modeling with GEE were very similar and therefore are omitted here. Figure 3 shows the observed and forecasted values of daily ED visits in the postsample forecasting set for the GLM and SARIMA models. As GLM and GEE results were almost identical, the latter was omitted for better visualization. The plots show that forecasted values successfully predicted the observed levels, as the lines representing each of those values follow a similar pattern, and there is an overlapping of values in the curves. Furthermore, all tested models could forecast major turning points in patient volume, i.e., the times at which upward and downward changes in observed values occurred were in agreement with changes in forecasted values. Comparison of graphs, including and without including temperature values, did not show significant differences. We calculated the MAPE of each model in order to compare their accuracy and decide which would be the most useful in our setting. Table 2 shows the calculated MAPEs of all tested models in the three postsample 30-days forecasting horizons. MAPEs are also shown for the first 7 days of each horizon. In general, MAPEs for the 7-day horizons were lower than for the 30-day horizons. Also, MAPEs decreased from first to third horizon. Calculated MAPEs from GLM and GEE were very similar and gave better results than SARIMA. Controlling for the effect of temperature did not improve ED patient visit forecasting accuracy. The calculated MAPEs from models including temperature values resulted in worse or similar forecasting ability. This study assessed different methods for forecasting daily ED visits and compared the accuracy of models with and without consideration of ambient temperature effects. We found that calendar variables were more important forecasting factors than ambient temperature. Moreover, our results showed that weekly seasonality was more dominant than monthly seasonality on daily ED patient visit variation throughout the study period, and Mondays presented the highest ED patient volumes while weekends presented the lowest. These results are in accordance with previous results.5, 9, 12, 14, 17 Our models could reliably predict patterns of daily ED visits. GLM and GEE models gave very similar results, and both showed better forecasting accuracy than SARIMA models. ARIMA models have been extensively used for health events forecasting purposes,3, 12, 17 although previous studies confirm our findings that other time-series methods perform equally to or better than ARIMA.7, 12 Tandberg and Qualls7 compared five different methods for forecasting hourly ED visits, including ARIMA, and found that a less complex moving average model had the best forecasting power. Jones et al.12 compared SARIMA, multiple linear regression, time-series regression, exponential smoothing, and artificial neural network models accuracy in forecasting daily ED volume at three facilities in the United States and found that time-series regression had the best and most consistent accuracy of all tested models. Besides their better accuracy in our study, we believe that for practical purposes GLM and GEE are advantageous over SARIMA, since fitting a SARIMA model is an iterative process and the autoregressive and moving average structures need to be reidentified as updated data are included in the training series, making it difficult to use it as an automated process. Seasonal control in previous studies was mostly done by the inclusion of categorical variables within selected time stratifications, e.g., indicator terms for each month of the year. This approach is disadvantageous, as it fails to control for seasonal changes within a month, and assumes a step change in events at the end of each period. We controlled for annual seasonal patterns using Fourier terms, which model regularly repeating seasonal cycles of different frequencies. Despite using this different approach, the overall MAPEs obtained from our models were in accordance with MAPEs published in previous studies. Reis and Mandl35 forecasted daily ED pediatric visits with ARIMA models and found a MAPE of 9.4%. Jones et al.12 found MAPE results that ranged from 8.5% to 15.5%. Sun et al.17 used a SARIMA model including terms for weather and air pollution to forecast daily ED visits at three Singapore health facilities and found MAPEs of 6.7, 8.6, and 16.9%. Kam et al.20 forecasted daily ED visits in a Korean hospital using both a univariate and a multivariate SARIMA model including weather variables, with resulting MAPEs of 7.8 and 7.4%, respectively. Boyle et al.13 forecasted daily ED presentations in two hospitals in Australia and obtained a MAPE of 7%. According to the MAPEs, the GLM and GEE were accurate and both models were better for the 7-day than the 30-day horizon. Moreover, MAPEs at the third horizon (December 2010) were lower than at the second (November 2010) and first (October 2010) horizons. This could possibly be due to the fact that the third horizon included a greater amount of data in the training set being regressed, thus resulting in more accurate forecasting of ED patient visits. Another possible explanation for such a result is that the third horizon may be a more predictable one, as December corresponds to school holidays, and a significant amount of people leave the city for summer vacations, Christmas, and New Year's Eve holidays. In all 3 years of the data set, December had the lowest number of observed ED visits. Our findings do not support including terms for ambient temperature effects in ED patient visit forecasting models. Simpler models based on calendar variables, i.e., models that did not include temperature variables for forecasting ED daily visits, performed equally to or better than the more complex ones. Besides not improving ED demand forecast accuracy, weather conditions cannot, of course, be forecast with 100% accuracy, especially for horizons of more than 3 days in advance, thus adding further inaccuracies to an ED patient visit forecast model. Moreover, ED patient visit forecasting models based solely on calendar variables are more easily set up as an automated process and can be provided well in advance, leaving enough time for administrative decisions. Although it may be expected that forecasting models of ED patient visits including terms for temperature effects would perform at least as well as those that did not include such terms, our results showing the contrary are not unusual. Chatfield11 argues that even though complex forecasting models in general give a better fit than simpler models (and this was actually true for our models), the resulting forecast is not necessarily more accurate, and simpler models are often better. The ultimate goal of this study was to explore models for predicting ED patient volume that health care managers can employ for better planning and resource allocation. To fulfill this, such models should be tested in practice. Although our models could successfully forecast the pattern of daily ED visits, there were some days that were associated with high absolute errors, which could hinder use of the forecasting method in real-case scenarios. On the other hand, previous studies with similar accuracy to our models reported promising results when used for managerial decisions. For instance, Batal et al.5 found an 18.5% decrease in patients leaving without being seen and a 30% decrease in complaints, after applying their model to inform staffing levels. The evaluation data set included only months of the warmest season in Brazil (October through December), so we could not evaluate how the model would perform during the winter period. Extending the database to forecast daily ED visits during the winter or other seasons to assess any changes in the models' forecast accuracy could be of great importance. Our models included those predictive variables that could be measured readily in our setting, but there may be other factors affecting daily ED visits that could not be evaluated in this study, such as the availability of other primary care facilities and their workload.9 Other environmental factors not considered here also have the potential to make a contribution to model performance. Our study indicates that time-series models can be developed to provide accurate short-range forecasts of ED patient visits, which might inform future ED staffing choices. Forecasting ability was dependent on the type of model employed and the length of the time horizon being predicted. In our setting, generalized linear models and generalized estimating equation models showed better accuracy than seasonal autoregressive integrated moving average models, and including information about ambient temperature did not improve forecasting accuracy. Although there were occasional days with relatively high forecast errors, forecasting models based on calendar variables alone did in general detect patterns of daily variability in ED volume, and thus could be used for developing an automated system for better planning of personnel resources.
Referência(s)