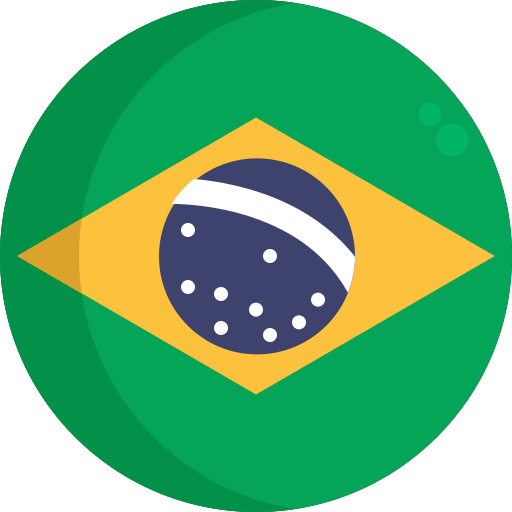
External Validation of Outcome Prediction Model for Ureteral/Renal Calculi
2006; Lippincott Williams & Wilkins; Volume: 175; Issue: 2 Linguagem: Inglês
10.1016/s0022-5347(05)00244-2
ISSN1527-3792
AutoresSijo Parekattil, Udaya Kumar, Nicholas J. Hegarty, Christopher S. Williams, Tara Allen, Patrick Telöken, Victor A. Leitão, Nelson Rodrígues Netto, Georges‐Pascal Haber, C. Ballereau, Arnauld Villers, Stevan B. Streem, Mark White, Michael E. Moran,
Tópico(s)Kidney Stones and Urolithiasis Treatments
ResumoNo AccessJournal of UrologyAdult urology1 Feb 2006External Validation of Outcome Prediction Model for Ureteral/Renal Calculiis accompanied byWhy Does the Bonn Risk Index Discriminate Between Calcium Oxalate Stone Formers and Healthy Controls? Sijo J. Parekattil, Udaya Kumar, Nicholas J. Hegarty, Clay Williams, Tara Allen, Patrick Teloken, Victor A. Leitão, Nelson R. Netto, Georges-Pascal Haber, Charles Ballereau, Arnauld Villers, Stevan B. Streem, Mark D. White, and Michael E. Moran Sijo J. ParekattilSijo J. Parekattil , Udaya KumarUdaya Kumar , Nicholas J. HegartyNicholas J. Hegarty , Clay WilliamsClay Williams , Tara AllenTara Allen , Patrick TelokenPatrick Teloken , Victor A. LeitãoVictor A. Leitão , Nelson R. NettoNelson R. Netto , Georges-Pascal HaberGeorges-Pascal Haber , Charles BallereauCharles Ballereau , Arnauld VillersArnauld Villers , Stevan B. StreemStevan B. Streem , Mark D. WhiteMark D. White , and Michael E. MoranMichael E. Moran View All Author Informationhttps://doi.org/10.1016/S0022-5347(05)00244-2AboutFull TextPDF ToolsAdd to favoritesDownload CitationsTrack CitationsPermissionsReprints ShareFacebookLinked InTwitterEmail Abstract Purpose: We externally validated a previously designed neural network model to predict outcome and duration of passage for ureteral/renal calculi. The model was also evaluated using a 6 mm largest stone dimension cutoff in predicting stone outcome. Materials and Methods: The model was previously designed on 301 patients at Albany Medical Center (free shareware from www.uroengineering.com). The model had a prediction accuracy of 86% for passage outcome and 87% for passage duration. In this study we tested the model on a separate 384 patients from 6 different external institutions to assess the prediction accuracy. All patients had a single renal/ureteral calculus by evaluation in an emergency room setting or by primary physicians and were then referred for further treatment. Model accuracy was also compared to using a 6 mm largest stone dimension cutoff in predicting the need for intervention. Results: Testing on the 384 patients from all 6 external institutions revealed an outcome prediction accuracy of 88%. The area under the ROC curve was 0.9. Using a 6 mm stone size cutoff provided 79% (ROC 0.8) accuracy. The model duration of passage prediction accuracy was 80% (133 patients passed the stone, area under ROC of 0.8). Conclusions: The model provided high stone outcome prediction accuracy (ROC of 0.9 and 0.8) at the 6 external institutions, comparable to that of the design institution. The model provided higher accuracy than using only the largest stone dimension as a cutoff. Increasing experience will further assess the model's accuracy. References 1 : Time to stone passage for observed ureteral calculi a guide for patient education. J Urol1999; 162: 688. Link, Google Scholar 2 : Relationship of spontaneous passage of ureteral calculi to stone size and location as revealed by unenhanced helical CT. AJR Am J Roentgenol2002; 178: 101. Google Scholar 3 : Ureterolithiasis: can clinical outcome be predicted with unenhanced helical CT?. Radiology1998; 208: 97. Google Scholar 4 : A computer model to predict the outcome and duration of ureteral or renal calculous passage. J Urol2004; 171: 1436. Abstract, Google Scholar 5 : . New York: S. Karger Publishers1996: 136. chapt. 11. Google Scholar 6 : Epidemiology, Biostatistics, and Preventive Medicine. Philadelphia: W. B. Saunders Co1996: 172. chapt. 13. Google Scholar 7 : Artificial neural networks opening the black box. Cancer2001; 91: 1615. Google Scholar 8 : Artificial neural networks for diagnosis and prognosis in prostate cancer. Semin Urol Oncol2002; 20: 89. Google Scholar Cleveland Clinic Foundation, Cleveland, Ohio, University of Arkansas for Medical Sciences, Little Rock, Arkansas, Fundação Faculdade Federal de Ciências Médicas, Porto Alegre, Universidade Estadual de Campinas, Sao Paulo, Brazil, Hopital Huriez, Lille, France, St. Peter's Medical Center and Albany Medical Center, Albany, New York© 2006 by American Urological AssociationFiguresReferencesRelatedDetailsRelated articlesJournal of Urology9 Nov 2018Why Does the Bonn Risk Index Discriminate Between Calcium Oxalate Stone Formers and Healthy Controls? Volume 175Issue 2February 2006Page: 575-579 Advertisement Copyright & Permissions© 2006 by American Urological AssociationKeywordskidney calculineural networks (computer)ureteral calculiMetricsAuthor Information Sijo J. Parekattil Nothing to disclose. More articles by this author Udaya Kumar Nothing to disclose. More articles by this author Nicholas J. Hegarty Nothing to disclose. More articles by this author Clay Williams Nothing to disclose. More articles by this author Tara Allen Nothing to disclose. More articles by this author Patrick Teloken Nothing to disclose. More articles by this author Victor A. Leitão Nothing to disclose. More articles by this author Nelson R. Netto Nothing to disclose. More articles by this author Georges-Pascal Haber Nothing to disclose. More articles by this author Charles Ballereau Nothing to disclose. More articles by this author Arnauld Villers Nothing to disclose. More articles by this author Stevan B. Streem Nothing to disclose. More articles by this author Mark D. White Financial interest and/or other relationship with Merck, Johnson & Johnson, Pfizer, Eli Lilly & Co., Schwarz Pharma and GlaxoSmithKline. More articles by this author Michael E. Moran Nothing to disclose. More articles by this author Expand All Advertisement PDF downloadLoading ...
Referência(s)