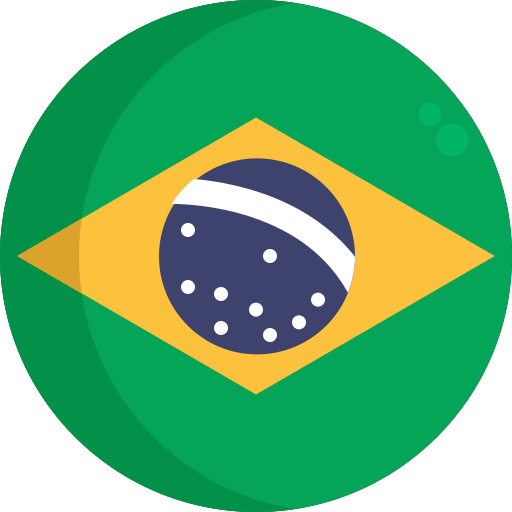
Optimal periodic maintenance policy under imperfect repair: A case study on the engines of off-road vehicles
2016; Taylor & Francis; Volume: 48; Issue: 8 Linguagem: Inglês
10.1080/0740817x.2016.1147663
ISSN1545-8830
AutoresMaria Luíza Guerra de Toledo, Marta Afonso Freitas, Enrico A. Colosimo, Gustavo L. Gilardoni,
Tópico(s)Software Reliability and Analysis Research
ResumoABSTRACTIn the repairable systems literature one can find a great number of papers that propose maintenance policies under the assumption of minimal repair after each failure (such a repair leaves the system in the same condition as it was just before the failure—as bad as old). This article derives a statistical procedure to estimate the optimal Preventive Maintenance (PM) periodic policy, under the following two assumptions: (i) perfect repair at each PM action (i.e., the system returns to the as-good-as-new state) and (ii) imperfect system repair after each failure (the system returns to an intermediate state between as bad as old and as good as new). Models for imperfect repair have already been presented in the literature. However, an inference procedure for the quantities of interest has not yet been fully studied. In the present article, statistical methods, including the likelihood function, Monte Carlo simulation, and bootstrap resampling methods, are used in order to (i) estimate the degree of efficiency of a repair and (ii) obtain the optimal PM check points that minimize the expected total cost. This study was motivated by a real situation involving the maintenance of engines in off-road vehicles.KEYWORDS: Reliabilitymaintenanceimperfect repairvirtual age modelgreatest convex minorantpower law processintensity functionoptimal stopping FundingThis work was partially supported by CNPq, FAPDF, and FAPEMIG grants.Additional informationNotes on contributorsMaria Luíza Guerra de ToledoMaria Luíza Guerra de Toledo is a Researcher and Professor at Escola Nacional de Ciências Estatísticas (Brazilian Institute of Geography and Statistics, Brazil). She has a bachelor's and a master's degree in Statistics from Universidade Federal de Minas Gerais–Brazil (2005,2007) and a Ph.D. in Industrial Engineering from the Universidade Federal de Minas Gerais (2014). Her research areas are probability and statistics applied to survival analysis, reliability, and maintenance.Marta A. FreitasMarta Afonso Freitas is an Associate Professor at the Universidade Federal de Minas Gerais, Brazil. She has a bachelor's degree in Statistics from UNICAMP–Brazil (1982), a master's degree in Statistics from the University of Wisconsin–Madison (1991), and a Ph.D. in Industrial Engineering from USP–Brazil (2000). She was a postdoctoral fellow at the Université de Technologie de Compiègne–França (2013–2014). Her research areas are reliability and maintenance (life tests and accelerated degradation tests and statistical models for equipment maintenance), and quantitative methods for product development.Enrico A. ColosimoEnrico Antônio Colosimo is the Titular Professor at the Universidade Federal de Minas Gerais, Brazil. Has a bachelor's degree in Electrical Engineering from the Universidade Federal de Minas Gerais (1981), a master's degree in Statistics from the Universidade Estadual de Campinas–Brazil (1985), and a Ph.D. in Statistics from the University of Wisconsin–Madison (1991). He was a postdoctoral fellow at IME-USP-Brazil (1996–1997) and Fundação Osvaldo Cruz, RJ, Brazil (2008–2009). His interest are in Statistics, with an emphasis on longitudinal data analysis, survival analysis, and reliability of repairable systems.Gustavo L. GilardoniGustavo Leonel Gilardoni Avalle is an Associate Professor at the Universidade de Brasília, Brazil. He has a master's degree in Estadistica Matemática from Centro Interamericano para la Enseñanza de La Estadística (CIENES, 1982) and a Ph.D in Statistics from the University of Wisconsin–Madison (1989). His research experience is centered on Probability and Statistics fields, with an emphasis on Bayesian inference, especially in the following themes: approximated Bayesian inference, hierarchical models, reliability of repairable systems, and information measures.
Referência(s)