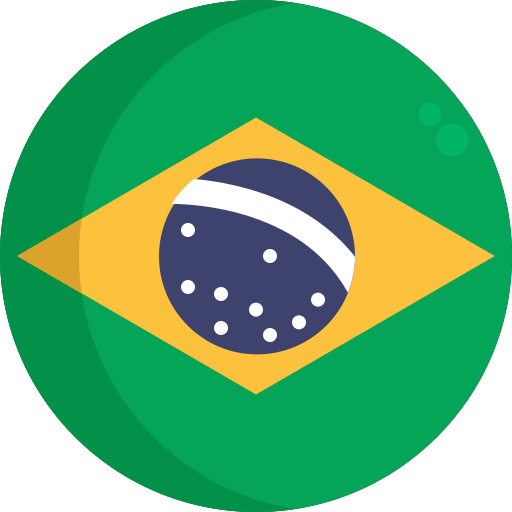
Impact of Tensor Cores and Mixed Precision on the Reliability of Matrix Multiplication in GPUs
2020; Institute of Electrical and Electronics Engineers; Volume: 67; Issue: 7 Linguagem: Inglês
10.1109/tns.2020.2977583
ISSN1558-1578
AutoresPedro Martins Basso, Fernando Fernandes dos Santos, Paolo Rech,
Tópico(s)Low-power high-performance VLSI design
ResumoMatrix multiplication (MxM) is a cornerstone application for both high-performance computing and safety-critical applications. Most of the operations in convolutional neural networks for object detection, in fact, are MxM related. Chip designers are proposing novel solutions to improve the efficiency of the execution of MxM. In this article, we investigate the impact of two novel architectures for MxM (i.e., tensor cores and mixed precision) on the graphics processing units (GPUs) reliability. In addition, we evaluate how effective the embedded error-correcting code is in reducing the MxM error rate. Our results show that low-precision operations are more reliable, and the tensor core increases the amount of data correctly produced by the GPU. However, reducing precision and the use of tensor core significantly increase the impact of faults in the output correctness.
Referência(s)