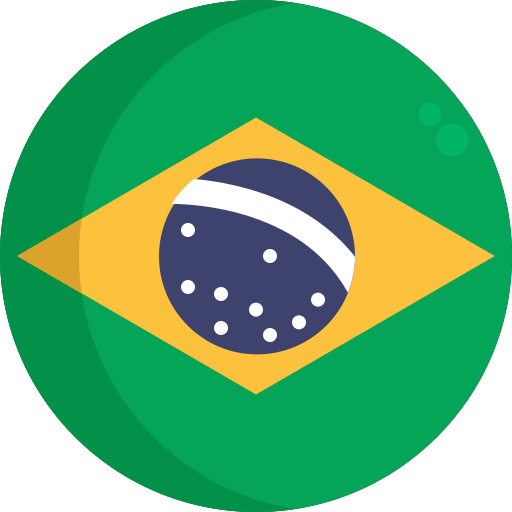
An enhanced set-membership evolving participatory learning with kernel recursive least squares applied to thermal modeling of power transformers
2020; Elsevier BV; Volume: 184; Linguagem: Inglês
10.1016/j.epsr.2020.106334
ISSN1873-2046
AutoresKaike Sa Teles Rocha Alves, Michel Hell, Fernando Luiz Cyrino Oliveira, Eduardo Pestana de Aguiar,
Tópico(s)Grey System Theory Applications
ResumoA factor that directly impacts the lifespan of a power transformer is the hot-spot temperature, and its monitoring is vital to prevent faults, reduce costs, keep the safety, and provide a reliable service to consumers. In this paper, we propose two forecasting models to predict the hot-spot temperature of power transformers. The first is the implementation of Set-Membership in the evolving Participatory Learning with Kernel Recursive Least Squares. And the second is a combination of the evolving Participatory Learning with Kernel Recursive Least Squares and the improved version of the Set-Membership concept, named Enhanced Set-Membership. Both Set-Membership and the Enhanced Set-Membership approaches are implemented to update the rate of change of the arousal index, which is a parameter that controls the creation of rules. A data set collected from an experimental transformer is adopted to evaluate the model’s performance. The obtained results are compared with the performance of the original evolving Participatory Learning with Kernel Recursive Least Squares and with the performance of other classical models suggested in the literature. The proposals have lower errors and a competitive number of final rules, suggesting that the models are efficient approaches to modeling complex data with high accuracy.
Referência(s)