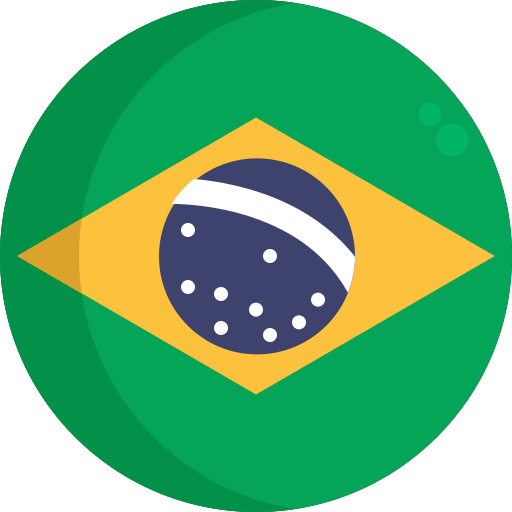
Deep Learning-Enhanced Internet of Medical Things to Analyze Brain CT Scans of Hemorrhagic Stroke Patients: A New Approach
2020; IEEE Sensors Council; Volume: 21; Issue: 22 Linguagem: Inglês
10.1109/jsen.2020.3032897
ISSN1558-1748
AutoresYongzhao Xu, Gabriel B. Holanda, Luís Fabrício de Freitas Souza, Hercules Silva, Adriell Gomes, I. G. M. e Silva, Marcos Vinícius Ferreira, Chuanyu Jia, Tao Han, Victor Hugo C. de Albuquerque, Pedro P. Rebouças Filho,
Tópico(s)COVID-19 diagnosis using AI
ResumoStroke is among the first pathologies that kill the most in the world, ranking second in deaths from illness. Each year, around 16 million people worldwide are victims of this disease, with approximately 38 % of cases brought to death. Computed Tomography (CT) is a super effective method to aid the medical diagnosis of stroke. But the analysis is subject to variations in the perception of specialists about its characteristics. Faced with the challenge of diagnosing stroke on CT images, this study proposes a fully automatic system based on Health of Things capable of classifying CT images of the skull through deep learning networks, classifying them into (Uninjured or Hemorrhagic stroke). After the image classification, Mask R-CNN segments the stroke through a learning transfer process, combined with machine learning methods. Our innovative method selected excellent results, both for classification with 100 % accuracy, and for segmentation in our best model (Mask + kNN) that reached 99.93% specificity and 99.73 accuracy, with segmentation time of 4.00 seconds, surpassing literature methods based on automatic models.
Referência(s)