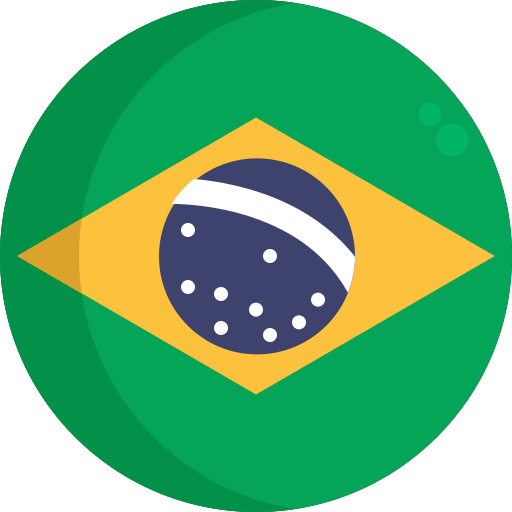
Q-Managed: A new algorithm for a multiobjective reinforcement learning
2020; Elsevier BV; Volume: 168; Linguagem: Inglês
10.1016/j.eswa.2020.114228
ISSN1873-6793
AutoresThiago Henrique Freire de Oliveira, Luiz Paulo de Souza Medeiros, Adrião Duarte Dória Neto, Jorge Dantas de Melo,
Tópico(s)Metaheuristic Optimization Algorithms Research
ResumoMulti-objective reinforcement learning (MORL) involves the use of reinforcement learning techniques to address problems with multiple objectives, conflicting or not. Among the main techniques used to treat this class of problems, we saw that they are limited by some factors, such as the Pareto Front shape and computational cost. This paper proposes a new iterative algorithm based on the single-policy approach, called Q-Managed. We use a hybrid multi-objective optimization (MOO) method that provides the mathematical guarantee that all policies belonging to the Pareto Front can be found, regardless of whether it is concave, convex or a mixture of both. Another important aspect that is worth mentioning is that its simplicity and performance are from a single-policy algorithms. To validate our proposal, we use the traditional MORL benchmarks and with different configurations of the Pareto Front. The Q-Managed shows success in finding all the optimal policies in all environments, surpassing all the single-policy algorithms in the literature in terms of policy quality. Based on the used benchmarks, its effectiveness can also be equated to the best multi-policy algorithms. The hypervolume metric was used to compare the quality of the policies found by our algorithm with those found in the state of the art. Extensions for non-episodic environments and stochastic transition functions are also introduced.
Referência(s)