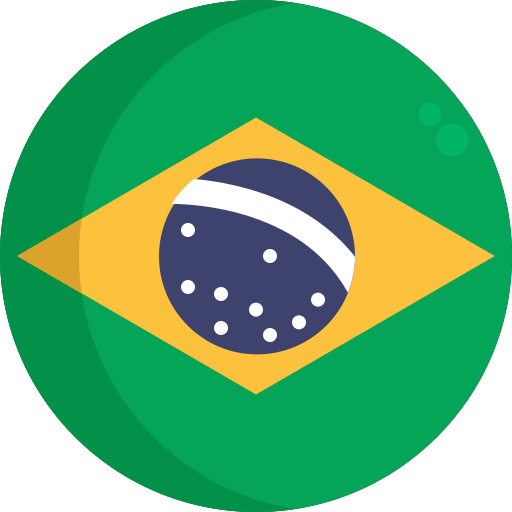
Evaluation of Docking Machine Learning and Molecular Dynamics Methodologies for DNA-Ligand Systems
2022; Multidisciplinary Digital Publishing Institute; Volume: 15; Issue: 2 Linguagem: Inglês
10.3390/ph15020132
ISSN1424-8247
AutoresTiago Alves de Oliveira, Lucas Rolim Medaglia, Eduardo Habib Bechelane Maia, Letícia C. Assis, Paulo Carvalho, Alisson Marques da Silva, Alex Gutterres Taranto,
Tópico(s)Advanced biosensing and bioanalysis techniques
ResumoDNA is a molecular target for the treatment of several diseases, including cancer, but there are few docking methodologies exploring the interactions between nucleic acids with DNA intercalating agents. Different docking methodologies, such as AutoDock Vina, DOCK 6, and Consensus, implemented into Molecular Architect (MolAr), were evaluated for their ability to analyze those interactions, considering visual inspection, redocking, and ROC curve. Ligands were refined by Parametric Method 7 (PM7), and ligands and decoys were docked into the minor DNA groove (PDB code: 1VZK). As a result, the area under the ROC curve (AUC-ROC) was 0.98, 0.88, and 0.99 for AutoDock Vina, DOCK 6, and Consensus methodologies, respectively. In addition, we proposed a machine learning model to determine the experimental ∆Tm value, which found a 0.84 R2 score. Finally, the selected ligands mono imidazole lexitropsin (42), netropsin (45), and N,N'-(1H-pyrrole-2,5-diyldi-4,1-phenylene)dibenzenecarboximidamide (51) were submitted to Molecular Dynamic Simulations (MD) through NAMD software to evaluate their equilibrium binding pose into the groove. In conclusion, the use of MolAr improves the docking results obtained with other methodologies, is a suitable methodology to use in the DNA system and was proven to be a valuable tool to estimate the ∆Tm experimental values of DNA intercalating agents.
Referência(s)