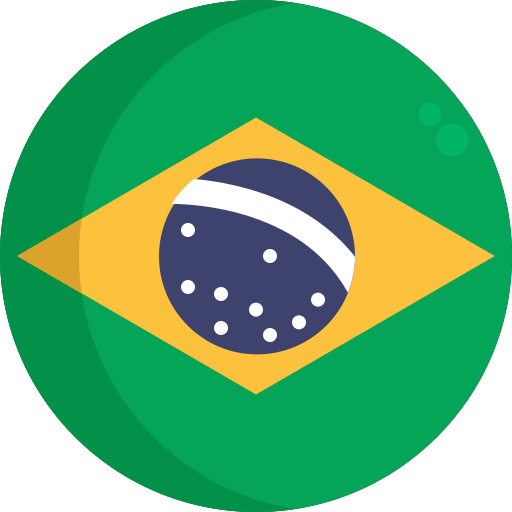
Glomerulosclerosis Identification Using a Modified Dense Convolutional Network
2022; Springer Science+Business Media; Linguagem: Inglês
10.1007/978-3-031-21686-2_17
ISSN1611-3349
AutoresJustino Santos, Vinícius Ponte Machado, Luciano Oliveira, Washington L. C. dos‐Santos, Nayze Lucena Sangreman Aldeman, Ângelo Duarte, Rodrigo Veras,
Tópico(s)Digital Imaging for Blood Diseases
ResumoGlomerulosclerosis is a common kidney disease characterized by the deposition of scar tissue, which replaces the renal parenchyma, and is quantified by renal pathologists to indicate the presence and extent of renal damage. It is paramount to guide the appropriate treatment and minimize the chances of the disease progressing to chronic stages. Thus, to identify glomerulus with sclerosis, this article proposes a convolutional neural network (CNN) inspired by convolutional blocks of DenseNet-201 but with smaller dense layers. We analyzed five CNNs - VGG-19, Inception-V3, ResNet-50, DenseNet-201, and EfficientNet-B2 - to define the best CNN model and evaluated several configurations for the fully connected layers. In total, 25 different models were analyzed. The experiments were carried out in three datasets, composed of 1,062 images, on which we applied data-augmentation techniques in the training set. These CNNs demonstrated effectiveness in the task and achieved an accuracy of 92.7% and kappa of 85.3%, considered excellent.
Referência(s)